Artificial intelligence (AI) is rapidly reshaping the landscape of modern medicine, stirring debates about its increasing role in clinical decision-making and patient management. At Bask Health, we recognize that while AI in Healthcare is revolutionizing medicine, its purpose is to complement—not replace—our healthcare professionals. With potential policy changes looming, such as the proposed amendments to the Federal Food, Drug, and Cosmetic Act (FDCA), including insights from House Bill 238, the intersection of AI in Healthcare and innovative technology is set to enter a new era.
In this comprehensive exploration, we examine the evolution of AI in Healthcare, its transformative impact on clinical workflows, and the revolutionary possibility of AI-driven drug prescriptions. We also discuss the current regulatory environment, ethical and legal considerations, and the myriad benefits and challenges ahead. Join us as we delve into the future of AI in Healthcare, and discover how emerging technologies are poised to work alongside medical professionals to enhance patient outcomes.
Key Takeaways
- AI is Transforming Healthcare, Not Replacing Doctors – AI in healthcare enhances clinical decision-making, automates administrative tasks, and personalizes patient care, but human oversight remains essential.
- AI-powered drug Prescriptions are Emerging – AI can analyze patient data, predict medication responses, and optimize treatment plans, potentially revolutionizing precision medicine.
- FDA is Reviewing AI’s Role in Healthcare – Regulatory changes, including House Bill 238, could classify AI as a medical device, ensuring oversight while accelerating adoption in healthcare.
- Ethical and Legal Challenges Persist – AI-driven decisions raise concerns about transparency, liability, and bias. Addressing these issues is key to responsible AI integration in healthcare.
- AI Boosts Efficiency and Cost Savings – Automating tasks like billing, scheduling, and diagnostics can save healthcare systems billions while improving patient outcomes.
- Remote Patient Monitoring and AI Diagnostics Are Expanding – AI enhances patient monitoring, reducing hospital visits and improving chronic disease management.
- Trust and Regulation Are Key to Adoption – AI must meet high safety standards, and healthcare providers, patients, and regulators must align to ensure its ethical and effective use.
The Rise of AI in Healthcare
Over the last decade, there has been an unprecedented rise in AI in Healthcare. From leveraging machine learning in Healthcare to integrating deep learning for diagnostic imaging, AI applications have become a cornerstone of modern medicine. Yet the most provocative question remains: Will AI in Healthcare replace doctors? The answer is a definitive no.
AI in Healthcare is engineered to support clinicians by providing robust data analysis, predictive insights, and personalized treatment plans. Its integration into daily practice aims to reduce administrative burdens, enhance diagnostic accuracy, and ultimately improve patient care outcomes. Moreover, emerging policy shifts—exemplified by discussions around the FDCA amendments—suggest that the FDA may soon authorize AI not only as a decision-support tool but potentially as a drug prescriber under strict regulatory oversight.
By aligning regulatory frameworks with the fast-evolving technology landscape, these changes are designed so AI in Healthcare maintains the highest standards of safety and efficacy, thereby enhancing the overall quality of care.
Understanding the Role of AI in Healthcare
Evolution of Artificial Intelligence in Medicine
The history of AI in Healthcare is a testament to technological evolution. In its early days, simple algorithms were employed to assist with basic diagnostic tasks and patient monitoring. Today, however, the scope of AI in Healthcare has expanded significantly:
- Early Adoption and Diagnostic Enhancements: Initially, AI systems were primarily used to analyze medical imaging and other diagnostic tests. With the advent of natural language processing (NLP) and sophisticated data analytics, AI now plays a critical role in interpreting vast quantities of electronic health record (EHR) data. This evolution has led to improved diagnostic accuracy and expedited clinical decision-making in AI in Healthcare.
- Machine Learning Advancements: The use of machine learning has allowed healthcare providers to refine treatment protocols and predict patient outcomes more accurately. For instance, studies like Schuitmaker et al. (2025) in the British Medical Bulletin emphasize the growing need for clinicians to master AI-assisted tools in clinical settings. These advancements are reshaping traditional approaches to disease diagnosis and treatment, reinforcing the value of precision medicine and personalized care within AI in Healthcare.
AI's Impact on Clinical Workflows
Integrating AI in Healthcare into clinical workflows has introduced transformative changes across several key areas:
- Streamlining Administrative Tasks: AI-powered systems are revolutionizing routine administrative functions such as scheduling, billing, and data entry. By automating these tasks, healthcare professionals can devote more time to patient care, thereby improving overall efficiency and patient satisfaction across the AI in the Healthcare spectrum.
- Clinical Decision Support: AI technologies are increasingly being employed as clinical decision support tools. By analyzing patient histories, imaging data, and laboratory results, AI can provide real-time recommendations to clinicians, reducing the likelihood of errors and enhancing diagnostic precision. This synergy between AI in Healthcare and human expertise is critical in areas such as drug discovery and personalized treatment planning.
- Patient Monitoring and Predictive Analytics: With the help of continuous data streams from wearable devices and remote monitoring systems, AI in Healthcare can track patient conditions and predict adverse events before they occur. This proactive approach to patient management is revolutionizing patient monitoring and opening new frontiers in preventive care.
AI Prescribing Drugs: A Paradigm Shift
How AI Could Prescribe Drugs
One of the most revolutionary applications of AI in Healthcare is its potential to prescribe drugs. Imagine a system that can analyze a patient’s entire health profile—integrating data from EHRs, genomic sequencing, and even lifestyle factors—to craft a personalized medication plan. This is the future of AI-powered Healthcare solutions:
- Data-Driven Analysis: Advanced AI algorithms are designed to sift through complex patient data, identifying patterns that human clinicians might overlook. For example, by evaluating genetic predispositions and environmental factors, AI can forecast a patient’s response to specific medications—showing how AI in Healthcare can be pivotal in tailoring treatments.
- Personalized Treatment Plans: By leveraging predictive analytics and machine learning in Healthcare, AI systems can tailor treatment plans to individual needs. This form of precision medicine not only improves therapeutic outcomes but also minimizes the risk of adverse drug reactions, a key benefit of integrating AI in Healthcare.
- Integration into Clinical Practice: In practical terms, AI-driven drug prescriptions would involve a collaborative process where AI provides recommendations that are then validated by clinicians. This partnership guarantees that the human element remains at the core of healthcare while benefiting from the efficiency and accuracy of AI in Healthcare.
A study by Pavon et al. (2025) in Diabetes Care highlights the promising role of AI in Healthcare in managing chronic diseases, demonstrating how AI and human intelligence can work in tandem to optimize patient outcomes.
Current Barriers and Limitations
Despite its transformative potential, the integration of AI in Healthcare for drug prescription is not without challenges:
- Ethical Concerns: Entrusting life-critical decisions to algorithms raises significant ethical questions. Trust, transparency, and accountability remain major hurdles. Ensuring that AI tools are free from bias and that their decision-making processes are transparent is essential for patient trust and safety in AI in Healthcare.
- Technical Challenges: While AI systems have advanced considerably, their accuracy and reliability must be continually validated. The complexity of human biology means that even small errors in an algorithm’s predictive model can have serious implications for patient health when relying on AI in Healthcare.
- Legal and Regulatory Hurdles: The regulatory landscape for AI in Healthcare is still evolving. Determining liability in cases of AI-related errors and navigating the approval processes for AI as a medical device poses significant challenges. Efforts to create a standardized framework for AI regulation in Healthcare are ongoing and essential to foster widespread adoption.
The Federal Food, Drug, and Cosmetic Act Amendment
Overview of the Proposed Amendments
Recent legislative discussions have brought House Bill 238 to the forefront, proposing critical amendments to the FDCA in response to the rapid evolution of healthcare technology, particularly in the realm of AI in Healthcare. These changes aim to create a regulatory environment that is better suited for the integration of advanced AI tools in medicine.
Key highlights of the proposed amendments include:
- Redefining Medical Devices: One of the cornerstone proposals is to classify certain AI technologies as medical devices. This would subject AI systems to rigorous FDA oversight similar to traditional medical equipment, ensuring that they meet established safety and efficacy standards in AI in Healthcare.
- Streamlined Approval Processes: Recognizing the iterative nature of AI development, the bill proposes more flexible regulatory pathways that can accommodate continuous updates and improvements without compromising patient safety—an essential step for the advancement of AI in Healthcare.
- Enhanced State-Level Authorization: The amendments also seek to harmonize state-level regulations by providing a more cohesive pathway for the integration of AI prescribers across different jurisdictions. This alignment is critical for ensuring consistent standards of care nationwide, a priority for AI in Healthcare.
Implications for AI in Healthcare
The proposed FDCA amendments could have far-reaching implications for the future of AI in Healthcare:
- Broader Adoption: By establishing clear regulatory guidelines, healthcare providers may gain greater confidence in deploying AI solutions. With a defined framework in place, clinicians can better integrate AI in Healthcare into their practice, knowing that these systems have undergone stringent evaluation.
- Increased Investment: Regulatory clarity can spur further investment in artificial intelligence in Healthcare research. As innovators and startups receive clearer signals from policymakers, the development of cutting-edge AI tools is likely to accelerate, further propelling AI in Healthcare.
- Enhanced Safety and Efficacy: With the FDA taking a more active role in overseeing AI technologies, the resulting safeguards will help ensure that these tools deliver on their promise of improved patient care while minimizing potential risks. For additional background on regulatory standards, check out the FDA's guidance on software as a medical device (SaMD).
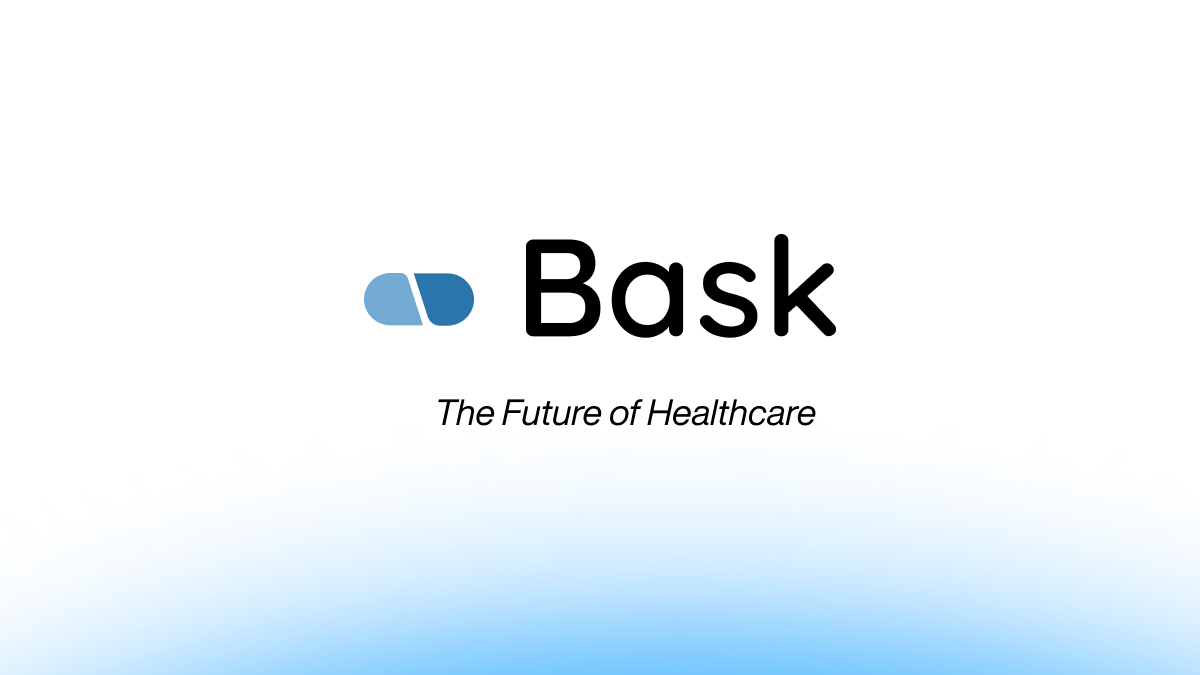
FDA Approval of AI Technologies
Current FDA Processes for AI
The U.S. Food and Drug Administration (FDA) has already begun to address the unique challenges posed by AI technologies in healthcare, particularly within AI in Healthcare. One of the key frameworks involves the regulation of software as a medical device (SaMD). This framework ensures that AI applications are subject to rigorous standards before they can be used in clinical settings.
- Premarket Notification (510(k)): This pathway requires manufacturers to demonstrate that their AI tool is substantially equivalent to an already approved device. By adhering to these guidelines, developers can bring innovative technologies to market more swiftly while ensuring the safety of AI in Healthcare.
- De Novo Classification: For novel AI systems without a direct predicate, the de novo pathway provides a mechanism for classification based on the device’s risk profile. This process allows for the introduction of groundbreaking technologies that do not fit neatly into existing categories, ensuring that innovation in AI in Healthcare is not stifled by outdated frameworks.
These pathways are designed to ensure that any AI tool used in healthcare not only meets rigorous performance standards but is also safe for patient use. As highlighted by Hswen and Rubin (2024) in the Journal of the American Medical Association, while AI-powered solutions show great promise, they must clear robust regulatory hurdles before achieving broad clinical acceptance in AI in Healthcare.
Future Directions
Looking forward, the regulatory landscape is expected to evolve in response to rapid technological advancements. Some of the key areas of development include:
- Adaptive Regulatory Models: AI systems are inherently dynamic, continuously learning and adapting. Future regulatory frameworks will need to accommodate these changes without compromising safety. This might involve regular updates to the AI's performance metrics and periodic re-evaluations to ensure ongoing efficacy in AI in Healthcare.
- Enhanced Collaboration: The future of AI in Healthcare will be shaped by closer collaboration between regulatory bodies, tech companies, and healthcare providers. Such partnerships will help bridge the gap between innovative technology and clinical practice, ensuring that AI tools are both cutting-edge and practical.
- Expanding Evaluation Metrics: New performance indicators tailored specifically to AI technologies—such as algorithmic transparency, bias mitigation, and adaptability—will likely become integral to the FDA’s evaluation process. This evolution is critical to ensure that AI in Healthcare not only meets traditional safety standards but also aligns with the ethical imperatives of modern medicine.
Ethical and Legal Considerations
Patient Safety and Data Privacy
As AI in Healthcare becomes more deeply integrated into everyday practice, concerns about patient safety and data privacy take center stage. The collection and analysis of large datasets are essential for the success of AI-driven systems; however, this also raises significant issues related to the protection of sensitive patient information.
- Data Security: Ensuring the security of electronic health records and other personal data is paramount. Robust encryption methods and secure data storage practices are necessary to protect against breaches that could compromise patient privacy in AI in Healthcare.
- Mitigating Bias: AI algorithms are only as effective as the data on which they are trained. Bias in data can lead to inaccurate or unfair outcomes, particularly in diverse patient populations. Strategies to address this include regular algorithm audits, the use of diverse training datasets, and the implementation of transparency measures in AI decision-making—crucial components of trustworthy AI in Healthcare.
- Patient Consent and Transparency: Patients must be fully informed about how their data will be used. Clear communication and robust consent protocols help build trust and ensure that patients understand the role of AI in their care.
For more detailed ethical guidelines, organizations such as the World Health Organization (WHO) provide comprehensive recommendations on the responsible use of AI in Healthcare.
Addressing Liability Issues
Determining accountability in an AI-driven healthcare system presents complex legal challenges:
- Responsibility in Case of Error: When an AI system makes a mistake that results in patient harm, the question of liability can become murky. Is the responsibility borne by the developer, the healthcare provider, or the institution that implemented the AI tool?
- Evolving Legal Precedents: The legal framework for AI in Healthcare is still in its early stages, with few precedents to guide decision-making. As cases involving AI-related malpractice emerge, it will be critical to establish clear guidelines for liability and compensation.
- Collaborative Accountability Models: Moving forward, a shared approach to accountability—where developers, clinicians, and institutions all bear responsibility—may be necessary. This collaborative model can help distribute risk and incentivize all parties to prioritize patient safety and ethical practice in AI in Healthcare.
Insights from the policy paper by Daneshvar et al. (2024) in Annals of Internal Medicine provide valuable perspectives on how liability issues might be navigated as AI becomes more prevalent in healthcare.
Benefits of AI in Healthcare in Drug Prescriptions
Enhanced Precision and Personalization
The promise of AI in Healthcare in drug prescriptions is perhaps most evident in its ability to deliver enhanced precision and personalization:
- Tailored Treatments: By leveraging detailed patient data—from genomic information to lifestyle factors—AI in Healthcare can help create highly individualized treatment plans. This approach is at the heart of precision medicine, ensuring that each patient receives the most effective medication for their unique profile.
- Reduction in Prescription Errors: AI-powered decision support systems minimize the risk of human error, ensuring accurate dosages and drug combinations. This improved diagnostic accuracy directly translates into better patient outcomes and increased trust in AI in Healthcare.
- Optimized Chronic Disease Management: For patients with long-term conditions such as diabetes, hypertension, or heart disease, AI in Healthcare can continuously monitor and adjust treatment protocols based on real-time data. This dynamic approach helps maintain optimal therapeutic levels and reduces complications over time.
Studies like the one by Pavon et al. (2025) underscore the synergy between AI in Healthcare and human expertise in managing chronic diseases, highlighting the significant benefits of personalized, data-driven treatment approaches.
Cost Efficiency and Scalability
Beyond clinical outcomes, the integration of AI in Healthcare into drug prescriptions offers significant economic advantages:
- Lower Healthcare Costs: Automating routine tasks and reducing human error can significantly lower overall healthcare costs. AI in Healthcare-driven efficiencies not only reduces expenditure but also streamlines operations, freeing up resources for more critical aspects of patient care.
- Scalability: AI in Healthcare solutions can be deployed across diverse healthcare settings—from large urban hospitals to remote rural clinics—ensuring that high-quality care is accessible to underserved populations. This scalability is crucial for bridging gaps in healthcare access and reducing disparities in treatment.
- Faster Access to Treatments: With AI in Healthcare systems processing and analyzing data in real-time, patients can benefit from quicker diagnosis and prompt initiation of the most appropriate therapy. This accelerated approach can be especially critical in emergency scenarios or for patients with rapidly evolving conditions.
The transformative potential of AI in Healthcare is evident in its ability to drive both clinical excellence and cost efficiency, making it a vital tool in the ongoing effort to modernize medicine.
Challenges Ahead
Gaining Trust Among Stakeholders
For AI in Healthcare to fully realize its potential in healthcare, it is imperative to build trust among all stakeholders:
- Clinicians and Medical Professionals: Many physicians are understandably cautious about relying on AI for critical decision-making. Ongoing education, transparent performance metrics, and robust clinical trials are needed to build confidence in these technologies. Initiatives that demonstrate the successful integration of machine learning in Healthcare can help shift perceptions and encourage wider adoption of AI in Healthcare.
- Patients: Patient skepticism remains a significant barrier. Clear, accessible explanations of how AI in Healthcare works and the safeguards in place to protect patient data are essential. Building a narrative that emphasizes AI in Healthcare as a supportive tool—rather than a replacement for the human touch—can help foster trust.
- Healthcare Institutions: Administrators and policymakers must be convinced of the long-term benefits of AI in Healthcare integration. Demonstrating improvements in cost efficiency, patient outcomes, and operational workflows is key to overcoming institutional resistance and driving systemic change.
A study by Traylor et al. (2025) in Cureus illustrates the importance of maintaining the patient-physician relationship as AI in Healthcare becomes more prevalent, underscoring the need for initiatives that foster trust and transparency.
Navigating State and Federal Regulations
The regulatory landscape for AI in Healthcare is complex and continuously evolving:
- Diverging State-Level Policies: With each state potentially adopting its own regulations regarding AI in Healthcare, healthcare providers may encounter a patchwork of standards that complicate implementation and interoperability.
- Need for Federal Standardization: A unified, federal framework for AI in Healthcare regulation is essential to ensure consistent standards of care across all jurisdictions. Efforts to align state policies with federal guidelines will help streamline compliance and promote innovation across the board.
- Ongoing Regulatory Adaptation: Given the rapid pace of technological change, regulators must remain agile. Collaborative efforts between state and federal agencies, along with continuous dialogue with industry experts, will be crucial in developing adaptable, forward-looking regulations that safeguard patient interests while promoting technological innovation in AI in Healthcare.
For more on regulatory trends, consider reviewing the FDA's digital health resources and publications from the National Institutes of Health (NIH).
In Conclusion
The advent of AI in Healthcare is not about replacing doctors but about empowering them with tools that enhance decision-making, improve patient care, and drive operational efficiency. AI’s potential to prescribe drugs represents just one facet of a broader transformation that includes improved diagnostics, personalized treatment plans, and streamlined clinical workflows.
As regulatory bodies like the FDA work to update policies in light of technological advances—exemplified by initiatives like House Bill 238—we must ensure that innovation proceeds hand in hand with patient safety, ethical standards, and robust legal frameworks. At Bask Health, we are committed to supporting ongoing research, fostering collaboration between technology innovators and clinicians, and advocating for regulations that protect both patients and healthcare providers—all while championing the advancements of AI in Healthcare.
The journey toward fully integrating AI in Healthcare into medicine is filled with promise, challenges, and opportunities. By embracing a balanced approach that marries the strengths of human expertise with the power of AI, we can unlock a future where healthcare is more precise, accessible, and effective for all.
FAQs
-
Q. What is the role of AI in drug prescription?
A. AI analyzes extensive patient data—including medical history, genetics, and environmental factors—to recommend personalized medication plans, thereby optimizing treatment outcomes within AI in Healthcare.
-
Q. How does the FDA regulate AI technologies?
A. The FDA employs frameworks such as software as a medical device (SaMD) and pathways like the 510(k) and de novo classifications to ensure that AI tools meet stringent safety and efficacy standards for AI in Healthcare.
-
Q. What are the main ethical concerns with AI prescribing drugs?
A. The primary concerns involve patient data privacy, algorithmic bias, and determining liability in the event of errors—issues that require transparency, rigorous testing, and collaborative oversight when implementing AI in Healthcare.
-
Q. Will AI replace doctors?
A. No, AI in Healthcare is designed to augment the capabilities of healthcare professionals by providing advanced analytics and decision support, while the human element remains crucial for empathy, judgment, and patient care.
-
Q. What is House Bill 238?
A. House Bill 238 is a proposed amendment to the FDCA intended to modernize regulatory oversight for emerging technologies, including AI in Healthcare, ensuring that they meet high standards of safety and efficacy.
References
- National Institutes of Health (NIH). Home. NIH. https://www.nih.gov/. Accessed February 13, 2025.
- Wikipedia. Natural language processing. Wikipedia. https://en.wikipedia.org/wiki/Natural_language_processing. Accessed February 13, 2025.
- Oxford Academic. AI in medical decision-making: A systematic review. British Medical Bulletin. https://academic.oup.com/bmb/article/153/1/ldae025/7958568. Accessed February 13, 2025.
- PubMed Central (PMC). AI applications in healthcare. PMC. https://pmc.ncbi.nlm.nih.gov/articles/PMC. Accessed February 13, 2025.
- American Diabetes Association. AI in diabetes management: Emerging trends. Diabetes Care. https://diabetesjournals.org/care/article/48/2/182/157738. Accessed February 13, 2025.
- U.S. Food and Drug Administration (FDA). Home. FDA. https://www.fda.gov/. Accessed February 13, 2025.
- Journal of the American Medical Association (JAMA). AI in clinical practice: Regulatory and ethical considerations. JAMA. https://jamanetwork.com/journals/jama/fullarticle/2828679. Accessed February 13, 2025.
- World Health Organization (WHO). Home. WHO. https://www.who.int/. Accessed February 13, 2025.
- Annals of Internal Medicine. AI-driven diagnostics: The evolving role of machine learning in medicine. Annals of Internal Medicine. https://www.acpjournals.org/doi/10.7326/M24-0146. Accessed February 13, 2025.
- American Diabetes Association. AI in diabetes management: Emerging trends. Diabetes Care. https://diabetesjournals.org/care/article/48/2/182/157738. Accessed February 13, 2025.
- National Center for Biotechnology Information (NCBI). Home. NCBI. https://www.ncbi.nlm.nih.gov/. Accessed February 13, 2025.
- Cureus. Beyond the screen: The impact of generative AI on patient learning and the physician-patient relationship. Cureus. https://www.cureus.com/articles/304273-beyond-the-screen-the-impact-of-generative-artificial-intelligence-ai-on-patient-learning-and-the-patient-physician-relationship#!/. Accessed February 13, 2025.
- U.S. Food and Drug Administration (FDA). Home. FDA. https://www.fda.gov/. Accessed February 13, 2025.
At Bask Health, we remain at the forefront of exploring how AI in Healthcare, machine learning in Healthcare, and other innovations are transforming the medical field. Stay tuned for more insights, expert analyses, and updates on the future of medicine as we continue to harness the potential of artificial intelligence to create a healthier tomorrow.